Fiber reinforced composites (such as carbon and glass) consist of long fibers oriented in a resin matrix. On the microscale (fiber/matrix) the position of fibers is random and subject to variability. Groups of fibers tend closer together to form fiber clusters and disperse to form matrix pockets. Microscale variability in fiber arrangement leads to variability in strength, often caused by stress concentrations forming between close fibers and low stiffness regions in matrix pockets. The problem is current modeling techniques fall short of capturing real life fiber arrangements, leading to less confidence in prediction of part performance under loading. This work aims at developing a robust method to characterize 2-D microscale fiber arrangements based on localized regions of fiber clusters and matrix pockets. With the aid of our NASA collaborators, this work can be integrated into an existing multiscale tool and used to create representative models to actual as-manufactured parts.
The characterization method was developed in MATLAB and works by reading in a microstructure and classifying it based on groups of close fibers (fiber clusters) or areas where there are little to no fibers (matrix-rich clusters). Cluster geometries are used as statistical descriptors to characterize each microstructure. In order to determine how these descriptors are made, a random RVE generator is used which has a range of inputs that control the final fiber positions. By randomly sampling these inputs and working with colleagues in the computer science department at UMass Lowell, machine learning models are being made to establish correlations between inputs and outputs. Finally, a reduced order model is being developed to simulate strength of randomly generated RVE’s. This method can be validated and verified with real scans of composite parts and research performed by collaborators on strength tests.
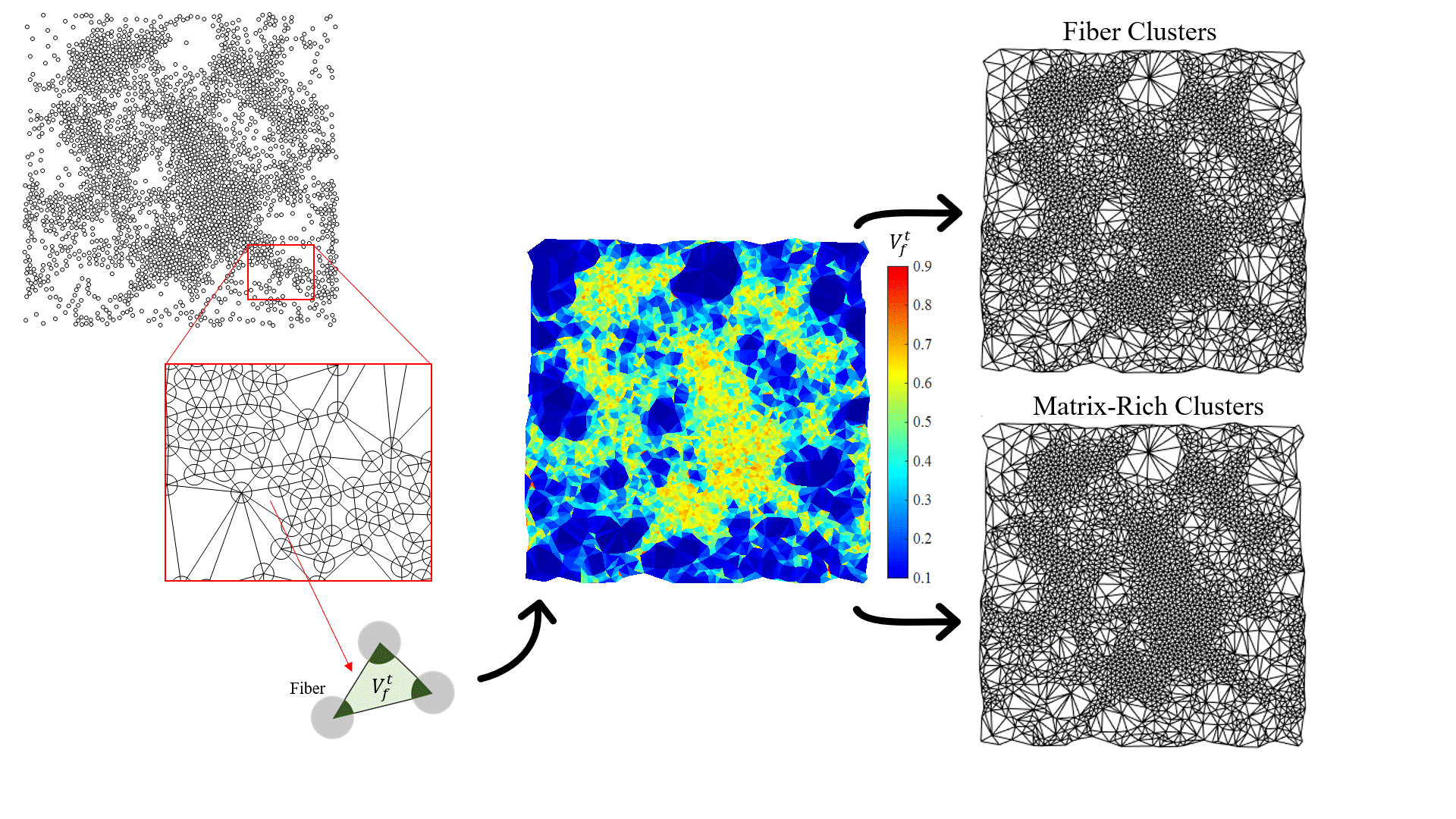